Note
Go to the end to download the full example code.
Fit Multiple Data Sets¶
Fitting multiple (simulated) Gaussian data sets simultaneously.
All minimizers require the residual array to be one-dimensional. Therefore, in
the objective
function we need to flatten
the array before returning it.
import matplotlib.pyplot as plt
import numpy as np
from lmfit import Parameters, minimize, report_fit
def gauss(x, amp, cen, sigma):
"""Gaussian lineshape."""
return amp * np.exp(-(x-cen)**2 / (2.*sigma**2))
def gauss_dataset(params, i, x):
"""Calculate Gaussian lineshape from parameters for data set."""
amp = params[f'amp_{i+1}']
cen = params[f'cen_{i+1}']
sig = params[f'sig_{i+1}']
return gauss(x, amp, cen, sig)
def objective(params, x, data):
"""Calculate total residual for fits of Gaussians to several data sets."""
ndata, _ = data.shape
resid = 0.0*data[:]
# make residual per data set
for i in range(ndata):
resid[i, :] = data[i, :] - gauss_dataset(params, i, x)
# now flatten this to a 1D array, as minimize() needs
return resid.flatten()
Create five simulated Gaussian data sets
np.random.seed(2021)
x = np.linspace(-1, 2, 151)
data = []
for _ in np.arange(5):
amp = 0.60 + 9.50*np.random.rand()
cen = -0.20 + 1.20*np.random.rand()
sig = 0.25 + 0.03*np.random.rand()
dat = gauss(x, amp, cen, sig) + np.random.normal(size=x.size, scale=0.1)
data.append(dat)
data = np.array(data)
Create five sets of fitting parameters, one per data set
Constrain the values of sigma to be the same for all peaks by assigning sig_2, …, sig_5 to be equal to sig_1.
for iy in (2, 3, 4, 5):
fit_params[f'sig_{iy}'].expr = 'sig_1'
Run the global fit and show the fitting result
[[Variables]]
amp_1: 6.32742010 +/- 0.02279089 (0.36%) (init = 0.5)
cen_1: 0.68049261 +/- 0.00126458 (0.19%) (init = 0.4)
sig_1: 0.25755570 +/- 4.9426e-04 (0.19%) (init = 0.3)
amp_2: 6.98604753 +/- 0.02296733 (0.33%) (init = 0.5)
cen_2: 0.50433700 +/- 0.00114536 (0.23%) (init = 0.4)
sig_2: 0.25755570 +/- 4.9426e-04 (0.19%) == 'sig_1'
amp_3: 7.11643510 +/- 0.02300415 (0.32%) (init = 0.5)
cen_3: -0.08260274 +/- 0.00112437 (1.36%) (init = 0.4)
sig_3: 0.25755570 +/- 4.9426e-04 (0.19%) == 'sig_1'
amp_4: 6.10197422 +/- 0.02273421 (0.37%) (init = 0.5)
cen_4: 0.07386098 +/- 0.00131130 (1.78%) (init = 0.4)
sig_4: 0.25755570 +/- 4.9426e-04 (0.19%) == 'sig_1'
amp_5: 9.23910555 +/- 0.02368872 (0.26%) (init = 0.5)
cen_5: 0.34443083 +/- 8.6605e-04 (0.25%) (init = 0.4)
sig_5: 0.25755570 +/- 4.9426e-04 (0.19%) == 'sig_1'
[[Correlations]] (unreported correlations are < 0.100)
C(sig_1, amp_5) = -0.3742
C(sig_1, amp_3) = -0.2968
C(sig_1, amp_2) = -0.2919
C(amp_1, sig_1) = -0.2664
C(sig_1, amp_4) = -0.2575
C(amp_3, amp_5) = +0.1111
C(amp_2, amp_5) = +0.1092
Plot the data sets and fits
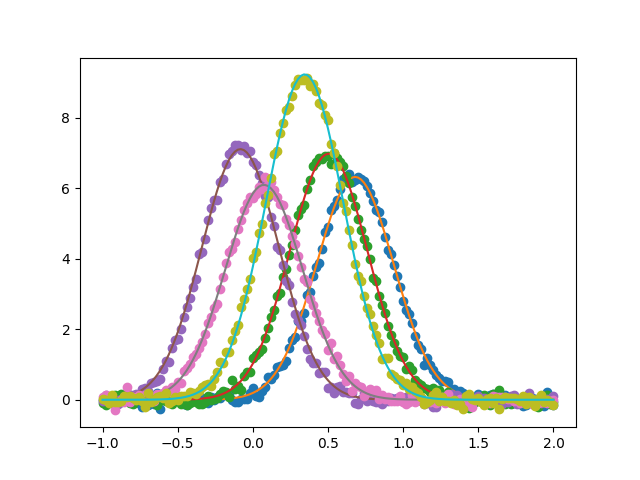
Total running time of the script: (0 minutes 0.341 seconds)